The variety and quantity of data available for clinical trials is greater than ever before. While this promises huge benefits in terms of monitoring subjects and measuring results, more information doesn’t always translate to more knowledge. Kumar Komuravelli, director of clinical data management at Mallinckrodt Pharmaceuticals, tells Grace Allen about the challenges facing clinical data management and how these developments can be capitalised upon.
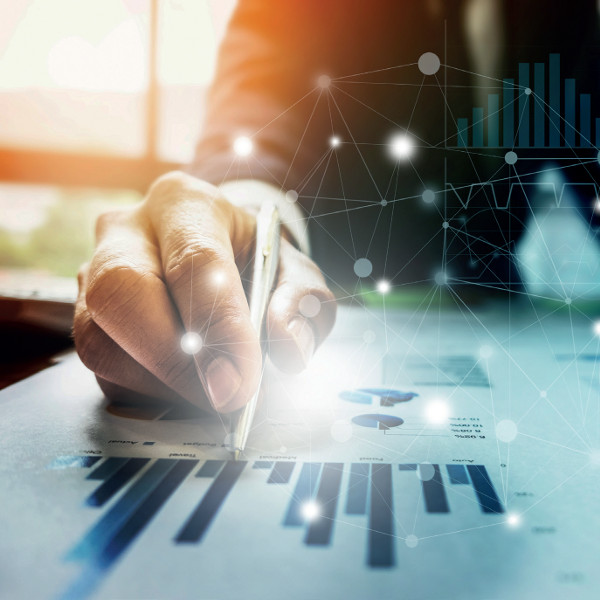
It is said that of all the data in the world, 90% originated in the past two years. According to the 2018 ‘Dojo Data Never Sleeps’ report, by 2020 1.7MB of data will be produced every second for every living person on earth. Every minute, over 3.8 million Google searches are made, nearly 160 million emails are sent and 473,400 tweets posted.
This avalanche of information is making itself felt in the conduct of clinical trials, offering huge potential for improvements and also putting increasing pressure on the management of clinical data. It is not only growing by volume: once largely limited to case report forms, the sources of clinical data are multiplying, with paper and electronic case report forms (CRFs) joined by electronic medical records (EMRs), electronic patient reported outcomes (ePRO) and a host of eSource data, captured straight from its electronic point of origin.
Predictions suggest that the proportion of non-CRF data will continue to rise. The ‘Tufts–Veeva 2017 eClinical Landscape Study’ found that, while eCRFs remain the source of the great majority of clinical trial data by volume (77.5%), the survey’s respondents expected their use of other sources to increase. 69.7% foresaw an increase in the overall number of sources they would make use of in the near future, while 91.6% predicted they would be using smartphone data in three years’ time, up from 44.8% incorporating it at present.
The sheer quantity of data points available from the development of internet of things (IoT)-enabled medical devices and applications brings opportunities and challenges. Smartphones, smartwatches, patches and other trackers can record variables such as heart rate, blood glucose level and blood pressure continuously and accurately, while eSource technology allows this to be collected in real time and with no potential errors arising from transcription. The use of wearables can streamline the conduct of a trial and extend its reach to wider demographics, and more data and greater data integrity give a clearer picture of a trial subject than ever before.
Not all data is useful data
However, just because vast wealth in terms of data points and volume is available doesn’t mean that it should all be collected.
“Do you really want to capture the blood pressure or heart rate every minute?” asks Kumar Komuravelli, director of clinical data management at Mallinckrodt Pharmaceuticals. “That’s a humungous amount of data. Even every hour – that’s still a lot of data you’re conducting to your trials. Is it useful?”
Komuravelli emphasises that the key to successful incorporation of IoT and eSource information is a strong understanding in the trial design phase of the purpose of each element.
“In data collection, the first question you need to ask is what you’re going to do with this data,” he says. “You need to have a road map of where you’re going to capture what data.”
In the end, collecting data that offers no value to the purpose of the trial creates unnecessary complexity.
Further complication is created by the disparate nature of much of the data now available, which can range from numerical values to medical images, genomic data and open text responses: these need significant manipulation to enable it to interact in a cohesive manner. A 2018 study carried out by Pharma Intelligence for Oracle Health Sciences found that over a quarter of respondents feared that the cost of conducting trials would be significantly increased due to the need to collect and manage new data types.
Clinical and metadata Repositories
The answer to this in the recent past has been the enterprise data warehouse, which combines data extracted from a range of sources together to allow for analysis. Now, however, data managers are combining or replacing this method with a clinical data repository (CDR), which allows the data to be used flexibly for multiple functions, or data lake solutions, which maintain the data in its original form.
By keeping the data in its source format, a data lake does not facilitate easy extraction of analytics and requires skilled analysts to interpret the information it contains. However, this approach reduces any errors or compromises that may arise from forcing disparate information into a proscribed record format, allows for greater detail to be preserved, and means that the requirements of the data can have flexibility.
In addition – and of great significance as the quantity of clinical trial data continues to rise – the data lake is a streamlined solution. While much time must be dedicated to the design of an enterprise data warehouse and to the entry of information from the original source, this is eliminated when it is kept in a copy of the original in a data lake.
As data multiplies, the role of metadata – the information that places each data point in its context – is vital. This is particularly true for a data lake or clinical data repository where well-defined metadata is needed to locate and analyse the contents. While metadata is another level of data to be managed, its creation can be automated and it can be housed in a metadata repository (MDR). Its use can lead to numerous advantages, one of which is for the review of data in order to develop future trials.
“It’s definitely useful,” Komuravelli says. “When you have these data structures – like MDR – developed, and then the actual clinical data is captured in a CDR model, it’s easy to pool the data, pull the data and review the data, and look at it from different angles for future studies for the companies. So what happened – why did this fail?”
In addition, metadata proves useful in the regulation of clinical trials. It makes each data point traceable throughout the trial lifetime, through any change in format, and means any request for information from a regulator is easy to comply with. Nevertheless, the cost of staying up to date can cause issues.
“This is an evolving technology regarding MDR from the past few years,” Komuravelli says. “The bigger companies have some rules and guidelines for what to do with it and how to use it, but I don’t think the midsize and smaller companies have that knowledge – the technology is expensive for smaller players.”
The impact of adaptive trial design
However, Komuravelli suggests that these companies can identify opportunities through collaboration, sharing knowledge on metadata processes to bring efficiencies into their work.
“Trying to use two different databases for two different cohorts is not the right thing to do; it’s a headache and it’s not the best practice,” Komuravelli says. “You need to consider the overall picture; what happens with the destination or study data page, how you want to capture the contents, what happens with the medical history and arrivals.
“You need to think, how can I design the study expecting that things will change over the period of the trial from cohort one to cohort two?”
Technological advances, such as artificial intelligence and machine learning facilitated by cloud computing and robotic automation offer avenues for managing this ocean of data, but the industry has to practice caution.
“The biotech industry moves slower in technology, the reason being that we are a regulated industry,” Komuravelli explains. “We’re constantly monitored by the regulatory authorities and we’re dealing with drugs: we’re conducting the clinical trials on patients, so you have to take this into serious consideration.”
Nevertheless, the industry is awake to the need to use state-of-the art methods to manage data.
“On one side there is the regulation, and on the other is the technology, but at the end of the day the technology wants to help you,” Komuravelli says. “As long as we know what we are doing, the technology definitely helps.”
One thing is clear: innovative, flexible and reliable data management techniques have never been more important.